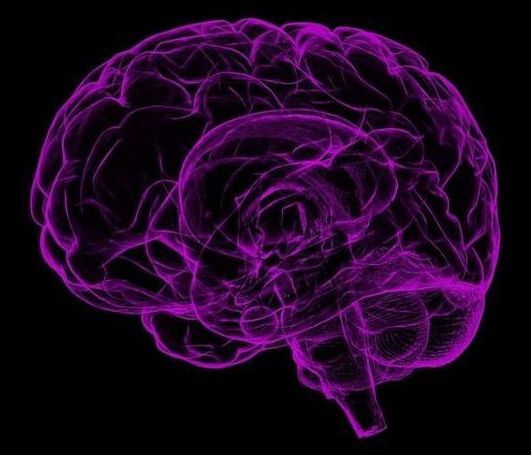
We all subconsciously learn complex behaviors in response to positive and negative feedback, but how that works in the brain remains a century-long mystery. By examining a powerful variant of reinforcement learning, dubbed distributional reinforcement learning, that outperforms original methods, the team suggests that the brain may simultaneously represent multiple predicted futures in parallel. Each future is assigned a different probability, or chance of actually occurring, based on reward.
Here’s the kicker: the team didn’t leave it as an AI-inspired hypothesis. In a collaboration with a lab at Harvard University, they recording straight from a mouse’s brain, and found signs of their idea encoded in its reward-processing neurons.