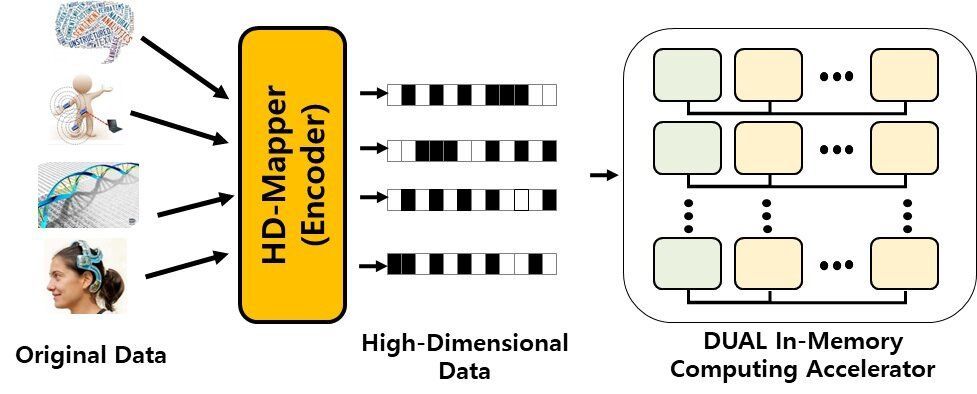
Scientists at DGIST in Korea, and UC Irvine and UC San Diego in the US, have developed a computer architecture that processes unsupervised machine learning algorithms faster, while consuming significantly less energy than state-of-the-art graphics processing units. The key is processing data where it is stored in computer memory and in an all-digital format. The researchers presented the new architecture, called DUAL, at the 2020 53rd Annual IEEE/ACM International Symposium on Microarchitecture.
âTodayâs computer applications generate a large amount of data that needs to be processed by machine learning algorithms,â says Yeseong Kim of Daegu Gyeongbuk Institute of Science and Technology (DGIST), who led the effort.
Powerful âunsupervisedâ machine learning involves training an algorithm to recognize patterns in large datasets without providing labeled examples for comparison. One popular approach is a clustering algorithm, which groups similar data into different classes. These algorithms are used for a wide variety of data analyzes, such as identifying fake news on social media, filtering spam email and detecting criminal or fraudulent activity online.