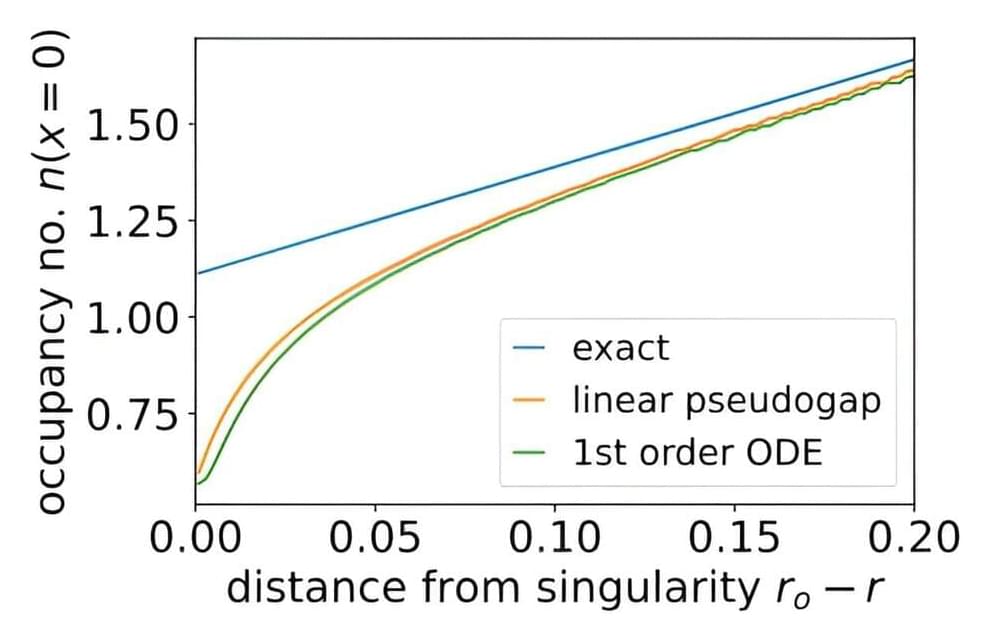
In Lewis Carroll’s Through the Looking-Glass, the Red Queen tells Alice, “It takes all the running you can do, to keep in the same place.” The race between innovation and obsolescence is like this.
Recent evidence about the slowing of technological and scientific progress in contrast to the accelerating epidemiological risks in a globalized world—in the opposite direction—indicates the importance of the relative rates of innovation and obsolescence.
When does innovation outpace, or fail to outpace, obsolescence? Understanding this dynamic is nascent, and the way that innovation is discussed is largely fragmented across fields. Despite some qualitative efforts to bridge this gap, insights are rarely transferred.