Podcast with “Andreessen Horowitz Distinguished Visiting Professor of Computer Science … Fei-Fei Li [who publishes under Li Fei-Fei], associate professor at Stanford University.”
Category: disruptive technology – Page 10
“So there came a time in which the ideas, although accumulated very slowly, were all accumulations not only of practical and useful things, but great accumulations of all types of prejudices, and strange and odd beliefs.
Then a way of avoiding the disease was discovered. This is to doubt that what is being passed from the past is in fact true, and to try to find out ab initio again from experience what the situation is, rather than trusting the experience of the past in the form in which it is passed down. And that is what science is: the result of the discovery that it is worthwhile rechecking by new direct experience, and not necessarily trusting the [human] race[’s] experience from the past. I see it that way. That is my best definition…Science is the belief in the ignorance of experts.“
–Richard P Feynman, What is Science? (1968)[1]
TruthSift.com is a platform designed to support and guide individuals or crowds to rationality, and make them smarter collectively than any unaided individual or group. (Free) Members use TruthSift to establish what can be established, refute what can’t be, and to transparently publish the demonstrations. Anyone can browse the demonstrations and learn what is actually known and how it was established. If they have a rational objection, they can post it and have it answered.
Whether in scientific fields such as climate change or medical practice, or within the corporate world or political or government debate, or on day to day factual questions, humanity hasn’t had a good method for establishing rational truth. You can see this from consequences we often fail to perceive:
Peer reviewed surveys agree: A landslide majority of medical practice is *not* supported by science [2,3,4]. Scientists are often confused about the established facts in their own field [5]. Within fields like climate science and vaccines, that badly desire consensus, no true consensus can be reached because skeptics raise issues that the majority brush aside without an established answer (exactly what Le Bon warned of more than 100 years ago[6]). Widely consulted sources like Wikipedia are reported to be largely paid propaganda on many important subjects [7], or the most popular answer rather than an established one [8]. Quora shows you the most popular individual answer, generated with little or no collaboration, and often there is little documentation of why you should believe it. Existing systems for crowd sourced wisdom largely compound group think, rather than addressing it. Existing websites for fact checking give you someone’s point of view.
Corporate or government planning is no better. Within large organizations, where there is inevitably systemic motivation to not pass bad news up, leadership needs active measures to avoid becoming clueless as to the real problems [9]. Corporate or government plans are subject to group think, or takeover by employee or other interests competing with the mission. Individuals who perceive mistakes have no recourse capable of rationally pursuading the majority, and may anyway be discouraged from speaking up by various consequences[6].
TruthSift is designed to solve all these problems. TruthSift realizes in your browser the Platonic ideal of the scientific literature, but TruthSift applies it to everything, and makes it tangible and lightweight, extended to a much lower hurdle for publishing. On a public TruthSift diagram, members (or on a Private diagram, members you have invited), who believe they can prove or refute a statement, can post their proof or refutation exactly where it is relevant. TruthSift logically propagates the consequences of each contribution, graphically displaying how it impacts the establishment status of all the others, drawing statements established by the combined efforts in thick borders, and statements refuted in thin. Statements are considered established only when they have an established demonstration, one with every posted challenge refuted.
is refuted. The statement menu is shown open in position to add a proof to this proof.
The topic statement is gold, pro statements are blue, con statements are red. Proof
connectors are black, challenges red, remarks purple, assumptions (not shown) blue.
Statements show the title. On the actual Topic the body can be seen by selecting
the statement and “View Statement” or hovering the mouse.
What is a proof? According to the first definition at Dictionary.com a proof is: “evidence sufficient to establish a thing as true, or to produce belief in its truth.” In mathematics, a proof is equivalent to a proof tree that starts at axioms, or previously established results, which the participants agree to stipulate, and proceeds by a series of steps that are individually unchallengeable. Each such step logically combines several conclusions previously established and/or axioms. The proof tree proceeds in this way until it establishes the stated proved conclusion. Mathematicians often raise objections to steps of the proof, but if it is subsequently established that all such objections are invalid, or if a workaround is found around the problem, the proof is accepted.
The Scientific literature works very similarly. Each paper adds some novel argument or evidence that previous work is true or is not true or extends it to establish new results. When people run out of valid, novel reasons why something is proved or is not proved, what remains is an established theory, or a refutation of it or of all its offered proofs.

Galileo’s: Dialogues Concerning the Two Chief World Views.
The black triangle indicates other incoming edges not shown. For complex diagrams,
it is often best to walk around in focused view centered on each statement in turn.
TruthSift is a platform for diagramming this process and applying it to any statements members care to propose to establish or refute. One may state a topic and add a proof tree for it, which is drawn as a diagram with every step and connection explicit. Members may state a demonstration of some conclusion they want to prove, building from some statements they assert are self-evident or that reference some authority they think trustworthy, and then building useful intermediate results that rationally follow from the assumptions, and building on until reaching the stated conclusion. If somebody thinks they find a hole in a proof at any step, or thinks one of the original assumptions need further proof, they can challenge it, explaining the problem they see. Then the writer of the proof (or others if its in collaboration mode) may edit the proof to fix the problem, or make clearer the explanation if they feel the challenger was simply mistaken, and may counter-challenge the challenge explaining that it had been resolved or mistaken. This can go on recursively, with someone pointing out a hole in the proof used by the counter-challenger that the challenge was invalid. On TruthSift the whole argument is laid out graphically and essentially block-chained, which should prevent the kind of edit-wars that happen for controversial topics on Wikipedia. Each challenge or post should state a novel reason, and when the rational arguments are exhausted, as in mathematics, what remains is either a proof of the conclusion or a refutation of it or all of its proofs.
As statements are added to a diagram, TruthSift keeps track of what is established and what refuted, drawing established statements’ borders and their outgoing connectors thick, and refuted statements’ borders and their outgoing connectors thin so viewers can instantly tell what is currently established and what refuted. TruthSift computes this by a simple algorithm that starts at statements with no incoming assumptions, challenges, or proofs, which are thus unchallenged as assertions that prove themselves, are self evident, or appeal to an authority everybody trusts. These are considered established. Then it walks up the diagram rating statements after all their parents have been rated. A statement will be established if all its assumptions are, none of its challenges are, and if it has proofs, at least one is established. (We support challenges requesting a proof be added to a statement which neither has one added nor adequately proves itself.) Otherwise, that is if a statement has an established challenge, or has refuted assumptions, or all of its proofs are refuted, it is refuted.
To understand why a statement is established or refuted, center focus on it, so that you see it and its parents in the diagram. If it is refuted, either there is an established challenge of it, or one of its assumptions is refuted, or all of its proofs are. If it is not refuted, it is established. Work your way backward up the diagram, centering on each statement in turn, and examine the reasons why it is established or refuted.

Fig 3: An example topic.
Effective contribution to TruthSift diagrams involves mental effort. This is both a hurdle and a feature. TruthSift teaches Critical Thinking. First you think about your Topic Statement. How actually should you specify Vaccine Safety or Climate Change, so it covers what you want to establish or refute, and so it is amenable to rational discussion? There is no place you could go to see that well specified now, and can you properly assure it without properly specifying it? Next you think about the arguments for your topic statement, and those against it, and those against the arguments for, and those for the arguments for, and the arguments against the arguments against, and so on until everybody runs out of arguments, when what is left is a concise rational analysis of what is established and why. The debate is settled point by point. The process naturally subdivides the field into sub-topics where different expertise’s come into play, promoting true collective wisdom and understanding.
For TruthSift to work properly, posters will have to respect the guidelines and post only proof or challenge statements that they believe rationally prove or refute their target and are novel to the diagram (or also novel additional evidence as assumptions or remarks or tests, which are alternative connector types). Posts violating the guidelines may be flagged and removed, and consistent violators as well. Posts don’t have to be correct, that’s what challenges are for, but they have to be honest attempts, not spam or ad hominem attacks. Don’t get hung up on whether a statement should be added as a proof or an assumption of another. Frequently you want to assemble arguments for a proposition stating something like “the preponderance of the evidence indicates X”, and these arguments are not individually necessary for X, nor are they individually proofs of X. It is safe to simply add them as proofs. They are not necessary assumptions, and if not enough of them are established, the target may be challenged on that basis. The goal is a diagram that transparently explains a proof and what is wrong with all the objections people have found plausible.
For cases where members disagree on underlying assumptions or basic principles, stipulation is available. If one or more statements are stipulated, statements are shown as conditionally true if established based on the stipulations and as conditionally false if refuted based on the stipulations. The challenges to the stipulation are also shown. TruthSift supports reasoning from different fundamental assumptions, but requires being explicit about it when challenged.
Probability mode supports the intuitive construction of probabilistic models, and evaluates the probability of each statement in the topic marginalizing over all the parameters in the topic. With a little practice these allow folding in various connections and evidence. These could be used for collaborative, verified, risk models; to support proofs with additional confidence tests; to reason about hidden causes; or many other novel applications

Rebut it if you can. Dashed edges represent citation into the literature. Title is shown for each
statement, on actual topic select “View Statement” to see body.
Basic Membership is free. In addition to public diagrams, debating the big public issues, private diagrams are available for personal or organizational planning or to exclude noise from your debate. Private diagrams have editing and/or viewing by invitation only. Come try it. http://TruthSift.com
TruthSift’s mission is to enable publication of a transparent exposition of human knowledge, so that anyone may readily determine what is truth and what fiction, what can be established by valid Demonstration and what can’t, and so that anyone can read and understand that Demonstration.
We intend the process of creating this exposition to lead to vastly increased understanding and improved critical thinking skills amongst our members and beyond. We hope to support collaborative human intelligences greater than any intelligence previously achieved on the planet, both in the public domain and for members’ private use.
And please, I’d love feedback or questions. [email protected]
1. Richard P Feynman, What is Science? (1968) http://www-oc.chemie.uni-regensburg.de/diaz/img_diaz/feynman…nce_68.pdf
2. Assessing the Efficacy and Safety of Medical Technologies, Office of Technology Assessment, Congress of the United States (1978)
http://www.fas.org/ota/reports/7805.pdf
3. Jeannette Ezzo, Barker Bausell, Daniel E. Moerman, Brian Berman and Victoria Hadhazy (2001). REVIEWING THE REVIEWS . International Journal of Technology Assessment in Health Care, 17, pp 457–466. http://journals.cambridge.org/action/displayAbstract?fromPag…aid=101041
4. John S Garrow BMJ. 2007 Nov 10; 335(7627): 951.doi:10.1136/bmj.39388.393970.1F PMCID: PMC2071976
What to do about CAM?: How much of orthodox medicine is evidence based?
http://www.dcscience.net/garrow-evidence-bmj.pdf
5. S. A. Greenberg, “How citation distortions create unfounded authority: analysis of a citation network”, BMJ 2009;339:b2680
http://www.bmj.com/content/339/bmj.b2680
6. Gustav Le Bon, The Crowd, (1895), (1995) Transaction Publishers New Edition Edition
7. S Attkisson, “Astroturf and manipulation of media messages”, TEDx University of Nevada, (2015) https://www.youtube.com/watch?v=-bYAQ-ZZtEU
8. Adam M. Wilson , Gene E. Likens, Content Volatility of Scientific Topics in Wikipedia: A Cautionary Tale 2015 DOI: 10.1371/journal.pone.0134454 http://journals.plos.org/plosone/article?id=10.1371/journal.pone.0134454
9. Kiira Siitari, Jim Martin & William W. Taylor (2014) Information Flow in Fisheries Management: Systemic Distortion within Agency Hierarchies, Fisheries, 39:6, 246–250, http://dx.doi.org/10.1080/03632415.2014.915814
Fox 29 — Good Day Philadelphia
http://www.fox29.com/140735577-video
NBC TV 10
http://www.nbcphiladelphia.com/news/local/Zombies-from-Phill…65101.html
CNN en Espanol
http://cnnespanol.cnn.com/video/cnnee-encuentro-intvw-joel-o…-cerebral/
If you’ve ever seen a “recommended item” on eBay or Amazon that was just what you were looking for (or maybe didn’t know you were looking for), it’s likely the suggestion was powered by a recommendation engine. In a recent interview, Co-founder of machine learning startup Delvv, Inc., Raefer Gabriel, said these applications for recommendation engines and collaborative filtering algorithms are just the beginning of a powerful and broad-reaching technology.
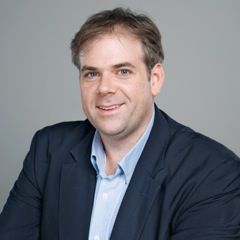
Gabriel noted that content discovery on services like Netflix, Pandora, and Spotify are most familiar to people because of the way they seem to “speak” to one’s preferences in movies, games, and music. Their relatively narrow focus of entertainment is a common thread that has made them successful as constrained domains. The challenge lies in developing recommendation engines for unbounded domains, like the internet, where there is more or less unlimited information.
“Some of the more unbounded domains, like web content, have struggled a little bit more to make good use of the technology that’s out there. Because there is so much unbounded information, it is hard to represent well, and to match well with other kinds of things people are considering,” Gabriel said. “Most of the collaborative filtering algorithms are built around some kind of matrix factorization technique and they definitely tend to work better if you bound the domain.”
Of all the recommendation engines and collaborative filters on the web, Gabriel cites Amazon as the most ambitious. The eCommerce giant utilizes a number of strategies to make item-to-item recommendations, complementary purchases, user preferences, and more. The key to developing those recommendations is more about the value of the data that Amazon is able to feed into the algorithm initially, hence reaching a critical mass of data on user preferences, which makes it much easier to create recommendations for new users.
“In order to handle those fresh users coming into the system, you need to have some way of modeling what their interest may be based on that first click that you’re able to extract out of them,” Gabriel said. “I think that intersection point between data warehousing and machine learning problems is actually a pretty critical intersection point, because machine learning doesn’t do much without data. So, you definitely need good systems to collect the data, good systems to manage the flow of data, and then good systems to apply models that you’ve built.”
Beyond consumer-oriented uses, Gabriel has seen recommendation engines and collaborative filter systems used in a narrow scope for medical applications and in manufacturing. In healthcare for example, he cited recommendations based on treatment preferences, doctor specialties, and other relevant decision-based suggestions; however, anything you can transform into a “model of relationships between items and item preferences” can map directly onto some form of recommendation engine or collaborative filter.
One of the most important elements that has driven the development of recommendation engines and collaborative filtering algorithms is the Netflix Prize, Gabriel said. The competition, which offered a $1 million prize to anyone who could design an algorithm to improve upon the proprietary Netflix’s recommendation engine, allowed entrants to use pieces of the company’s own user data to develop a better algorithm. The competition spurred a great deal of interest in the potential applications of collaborative filtering and recommendation engines, he said.
In addition, relative ease of access to an abundant amount of cheap memory is another driving force behind the development of recommendation engines. An eCommerce company like Amazon with millions of items needs plenty of memory to store millions of different of pieces of item and correlation data while also storing user data in potentially large blocks.
“You have to think about a lot of matrix data in memory. And it’s a matrix, because you’re looking at relationships between items and other items and, obviously, the problems that get interesting are ones where you have lots and lots of different items,” Gabriel said. “All of the fitting and the data storage does need quite a bit of memory to work with. Cheap and plentiful memory has been very helpful in the development of these things at the commercial scale.”
Looking forward, Gabriel sees recommendation engines and collaborative filtering systems evolving more toward predictive analytics and getting a handle on the unbounded domain of the internet. While those efforts may ultimately be driven by the Google Now platform, he foresees a time when recommendation-driven data will merge with search data to provide search results before you even search for them.
“I think there will be a lot more going on at that intersection between the search and recommendation space over the next couple years. It’s sort of inevitable,” Gabriel said. “You can look ahead to what someone is going to be searching for next, and you can certainly help refine and tune into the right information with less effort.”
While “mind-reading” search engines may still seem a bit like science fiction at present, the capabilities are evolving at a rapid pace, with predictive analytics at the bow.
How could global economic inequality survive the onslaught of synthetic organisms, micromanufacturing devices, additive manufacturing machines, nano-factories?
(http://www.beliefnet.com/columnists/lordre/2016/04/obsessed-…L36KMDo.99)
Narrated by Harry J. Bentham, author of Catalyst: A Techno-Liberation Thesis (2013), using the introduction from that book as a taster of the audio version of the book in production. (http://www.clubof.info/2016/04/liberation-technologies-to-come.html)
Paperback: http://www.amazon.com/Catalyst-Techno-Liberation-Harry-J-Ben…atfound-20
Kindle: http://www.amazon.com/Catalyst-Techno-Liberation-Harry-J-Ben…atfound-20
Audio: coming soon!
Bioquark, Inc., (http://www.bioquark.com) a company focused on the development of novel biologics for complex regeneration and disease reversion, and Revita Life Sciences, (http://revitalife.co.in) a biotechnology company focused on translational therapeutic applications of autologous stem cells, have announced that they have received IRB approval for a study focusing on a novel combinatorial approach to clinical intervention in the state of brain death in humans.
This first trial, within the portfolio of Bioquark’s Reanima Project (http://www.reanima.tech) is entitled “Non-randomized, Open-labeled, Interventional, Single Group, Proof of Concept Study With Multi-modality Approach in Cases of Brain Death Due to Traumatic Brain Injury Having Diffuse Axonal Injury” (https://clinicaltrials.gov/ct2/show/NCT02742857?term=bioquark&rank=1), will enroll an initial 20 subjects, and be conducted at Anupam Hospital in Rudrapur, Uttarakhand India.
“We are very excited about the approval of our protocol,” said Ira S. Pastor, CEO, Bioquark Inc. “With the convergence of the disciplines of regenerative biology, cognitive neuroscience, and clinical resuscitation, we are poised to delve into an area of scientific understanding previously inaccessible with existing technologies.”
Death is defined as the termination of all biological functions that sustain a living organism. Brain death, the complete and irreversible loss of brain function (including involuntary activity necessary to sustain life) as defined in the 1968 report of the Ad Hoc Committee of the Harvard Medical School, is the legal definition of human death in most countries around the world. Either directly through trauma, or indirectly through secondary disease indications, brain death is the final pathological state that over 60 million people globally transfer through each year.
While human beings lack substantial regenerative capabilities in the CNS, many non-human species, such as amphibians, planarians, and certain fish, can repair, regenerate and remodel substantial portions of their brain and brain stem even after critical life-threatening trauma.
Additionally, recent studies on complex brain regeneration in these organisms, have highlighted unique findings in relation to the storage of memories following destruction of the entire brain, which may have wide ranging implications for our understanding of consciousness and the stability of memory persistence.
“Through our study, we will gain unique insights into the state of human brain death, which will have important connections to future therapeutic development for other severe disorders of consciousness, such as coma, and the vegetative and minimally conscious states, as well as a range of degenerative CNS conditions, including Alzheimer’s and Parkinson’s disease,” said Dr. Sergei Paylian, Founder, President, and Chief Science Officer of Bioquark Inc.
Over the years, clinical science has focused heavily on preventing such life and death transitions and made some initial progress with suspended animation technologies, such as therapeutic hypothermia. However, once humans transition through the brain death window, currently defined by the medical establishment as “irreversible”, they are technically no longer alive, despite the fact that human bodies can still circulate blood, digest food, excrete waste, balance hormones, grow, sexually mature, heal wounds, spike a fever, and gestate and deliver a baby. It is even acknowledged by thought leaders that recently brain dead humans still may have residual blood flow and electrical nests of activity in their brains, just not enough to allow for an integrated functioning of the organism as a whole.
“We look forward to working closely with Bioquark Inc. on this cutting edge clinical initiative,” said Dr. Himanshu Bansal, Managing Director of Revita Life Sciences.
About Bioquark, Inc.
Bioquark Inc. is focused on the development of natural biologic based products, services, and technologies, with the goal of curing a wide range of diseases, as well as effecting complex regeneration. Bioquark is developing both biological pharmaceutical candidates, as well as products for the global consumer health and wellness market segments.
About Revita Life Sciences
Revita Life Sciences is a biotechnology company focused on the development of stem cell therapies that target areas of significant unmet medical need. Revita is led by Dr. Himanshu Bansal MD, PhD. who has spent over two decades developing novel MRI based classifications of spinal cord injuries as well as comprehensive treatment protocols with autologous tissues including bone marrow stem cells, dural nerve grafts, nasal olfactory tissues, and omental transposition.
“Virtually all new fossil fuel-burning power-generation capacity will end up “stranded”. This is the argument of a paper by academics at Oxford university. We have grown used to the idea that it will be impossible to burn a large portion of estimated reserves of fossil fuels if the likely rise in global mean temperatures is to be kept below 2C. But fuels are not the only assets that might be stranded. A similar logic can be applied to parts of the capital stock.”
“He is not here; He has risen,” — Matthew 28:6
As billions of Christians around the world are getting ready to celebrate the Easter festival and holiday, we take pause to appreciate the awe inspiring phenomena of resurrection.
In religious and mythological contexts, in both Western and Eastern societies, well known and less common names appear, such as Attis, Dionysus, Ganesha, Krishna, Lemminkainen, Odin, Osiris, Persephone, Quetzalcoatl, and Tammuz, all of whom were reborn again in the spark of the divine.
In the natural world, other names emerge, which are more ancient and less familiar, but equally fascinating, such as Deinococcus radiodurans, Turritopsis nutricula, and Milnesium tardigradum, all of whose abilities to rise from the ashes of death, or turn back time to start life again, are only beginning to be fully appreciated by the scientific world.
In the current era, from an information technology centric angle, proponents of a technological singularity and transhumanism, are placing bets on artificial intelligence, virtual reality, wearable devices, and other non-biological methods to create a future connecting humans to the digital world.
This Silicon Valley, “electronic resurrection” model has caused extensive deliberation, and various factions to form, from those minds that feel we should slow down and understand the deeper implications of a post-biologic state (Elon Musk, Steven Hawking, Bill Gates, the Vatican), to those that are steaming full speed ahead (Ray Kurzweil / Google) betting that humans will shortly be able to “transcend the limitations of biology”.
However, deferring an in-depth Skynet / Matrix discussion for now, is this debate clouding other possibilities that we have forgotten about, or may not have even yet fully considered?
Today, we find ourselves at an interesting point in history where the disciplines of regenerative sciences, evolutionary medicine, and complex systems biology, are converging to give us an understanding of the cycle of life and death, orders of magnitude more complex than only a few years ago.
In addition to the aforementioned species that are capable of biologic reanimation and turning back time, we show no less respect for those who possess other superhuman capabilities, such as magnetoreception, electrosensing, infrared imaging, and ultrasound detection, all of which nature has been optimizing over hundreds of millions of years, and which provide important clues to the untapped possibilities that currently exist in direct biological interfaces with the physical fabric of the universe.
The biologic information processing occurring in related aneural organisms and multicellular colony aggregators, is no less fascinating, and potentially challenges the notion of the brain as the sole repository of long-term encoded information.
Additionally, studies on memory following the destruction all, or significant parts of the brain, in regenerative organisms such as planarians, amphibians, metamorphic insects, and small hibernating mammals, have wide ranging implications for our understanding of consciousness, as well as to the centuries long debate between the materialists and dualists, as to whether we should focus our attention “in here”, or “out there”.
I am not opposed to studying either path, but I feel that we have the potential to learn a lot more about the topic of “out there” in the very near future.
The study of brain death in human beings, and the application of novel tools for neuro-regeneration and neuro-reanimation, for the first time offer us amazing opportunities to start from a clean slate, and answer questions that have long remained unanswered, as well as uncover a knowledge set previously thought unreachable.
Aside from a myriad of applications towards the range of degenerative CNS indications, as well as disorders of consciousness, such work will allow us to open a new chapter related to many other esoteric topics that have baffled the scientific community for years, and fallen into the realm of obscure curiosities.
From the well documented phenomena of terminal lucidity in end stage Alzheimer’s patients, to the mysteries of induced savant syndrome, to more arcane topics, such as the thousands of cases of children who claim to remember previous lives, by studying death, and subsequently the “biotechnological resurrection” of life, we can for the first time peak through the window, and offer a whole new knowledge base related to our place, and our interaction, with the very structure of reality.
We are entering a very exciting era of discovery and exploration.
About the author
Ira S. Pastor is the Chief Executive Officer of Bioquark Inc. (www.bioquark.com), an innovative life sciences company focusing on developing novel biologic solutions for human regeneration, repair, and rejuvenation. He is also on the board of the Reanima Project (www.reanima.tech)
At least in public relations terms, transhumanism is a house divided against itself. On the one hand, there are the ingenious efforts of Zoltan Istvan – in the guise of an ongoing US presidential bid — to promote an upbeat image of the movement by focusing on human life extension and other tech-based forms of empowerment that might appeal to ordinary voters. On the other hand, there is transhumanism’s image in the ‘serious’ mainstream media, which is currently dominated by Nick Bostrom’s warnings of a superintelligence-based apocalypse. The smart machines will eat not only our jobs but eat us as well, if we don’t introduce enough security measures.
Of course, as a founder of contemporary transhumanism, Bostrom does not wish to stop artificial intelligence research, and he ultimately believes that we can prevent worst case scenarios if we act now. Thus, we see a growing trade in the management of ‘existential risks’, which focusses on how we might prevent if not predict any such tech-based species-annihilating prospects. Nevertheless, this turn of events has made some observers reasonably wonder whether indeed it might not be better simply to put a halt to artificial intelligence research altogether. As a result, the precautionary principle, previously invoked in the context of environmental and health policy, has been given a new lease on life as generalized world-view.
The idea of ‘existential risk’ capitalizes on the prospect of a very unlikely event that, were it to pass, would be extremely catastrophic for the human condition. Thus, the high value of the outcome psychologically counterbalances its low probability. It’s a bit like Pascal’s wager, whereby the potentially negative consequences of you not believing in God – to wit, eternal damnation — rationally compels you to believe in God, despite your instinctive doubts about the deity’s existence.
However, this line of reasoning underestimates both the weakness and the strength of human intelligence. On the one hand, we’re not so powerful as to create a ‘weapon of mass destruction’, however defined, that could annihilate all of humanity; on the other, we’re not so weak as to be unable to recover from whatever errors of design or judgement that might be committed in the normal advance of science and technology in the human life-world. I make this point not to counsel complacency but to question whether ‘existential risk’ is really the high concept that it is cracked up to be. I don’t believe it is.
In fact, we would do better to revisit the signature Cold War way of thinking about these matters, which the RAND Corporation strategist Herman Kahn dubbed ‘thinking the unthinkable’. What he had in mind was the aftermath of a thermonuclear war in which, say, 25–50% of the world’s population is wiped out over a relatively short period of time. How do we rebuild humanity under those circumstances? This is not so different from ‘the worst case scenarios’ proposed nowadays, even under conditions of severe global warming. Kahn’s point was that we need now to come up with the relevant new technologies that would be necessary the day after Doomsday. Moreover, such a strategy was likely to be politically more tractable than trying actively to prevent Doomsday, say, through unilateral nuclear disarmament.
And indeed, we did largely follow Kahn’s advice. And precisely because Doomsday never happened, we ended up in peacetime with the riches that we have come to associate with Silicon Valley, a major beneficiary of the US federal largesse during the Cold War. The internet was developed as a distributed communication network in case the more centralized telephone system were taken down during a nuclear attack. This sort of ‘ahead of the curve’ thinking is characteristic of military-based innovation generally. Warfare focuses minds on what’s dispensable and what’s necessary to preserve – and indeed, how to enhance that which is necessary to preserve. It is truly a context in which we can say that ‘necessity is the mother of invention’. Once again, and most importantly, we win even – and especially – if Doomsday never happens.
An interesting economic precedent for this general line of thought, which I have associated with transhumanism’s ‘proactionary principle’, is what the mid-twentieth century Harvard economic historian Alexander Gerschenkron called ‘the relative advantage of backwardness’. The basic idea is that each successive nation can industrialise more quickly by learning from its predecessors without having to follow in their footsteps. The ‘learning’ amounts to innovating more efficient means of achieving and often surpassing the predecessors’ level of development. The post-catastrophic humanity would be in a similar position to benefit from this sense of ‘backwardness’ on a global scale vis-à-vis the pre-catastrophic humanity.
Doomsday scenarios invariably invite discussions of our species’ ‘resilience’ and ‘adaptability’, but these terms are far from clear. I prefer to start with a distinction drawn in cognitive archaeology between ‘reliable’ and ‘maintainable’ artefacts. Reliable artefacts tend to be ‘overdesigned’, which is to say, they can handle all the anticipated forms of stress, but most of those never happen. Maintainable artefacts tend to be ‘underdesigned’, which means that they make it easy for the user to make replacements when disasters strike, which are assumed to be unpredictable.
In a sense, ‘resilience’ and ‘adaptability’ could be identified with either position, but the Cold War’s proactionary approach to Doomsday suggests that the latter would be preferable. In other words, we want a society that is not so dependent on the likely scenarios – including the likely negative ones — that we couldn’t cope in case a very unlikely, very negative scenario comes to pass. Recalling US Defence Secretary Donald Rumsfeld’s game-theoretic formulation, we need to address the ‘unknown unknowns’, not merely the ‘known unknowns’. Good candidates for the relevant ‘unknown unknowns’ are the interaction effects of relatively independent research and societal trends, which while benign in themselves may produce malign consequences — call them ‘emergent’, if you wish.
It is now time for social scientists to present both expert and lay subjects with such emergent scenarios and ask them to pinpoint their ‘negativity’: What would be potentially lost in the various scenarios which would be vital to sustain the ‘human condition’, however defined? The answers would provide the basis for future innovation policy – namely, to recover if not strengthen these vital features in a new guise. Even if the resulting innovations prove unnecessary in the sense that the Doomsday scenarios don’t come to pass, nevertheless they will make our normal lives better – as has been the long-term effect of the Cold War.
References
Bleed, P. (1986). ‘The optimal design of hunting weapons: Maintainability or reliability?’ American Antiquity 51: 737– 47.
Bostrom, N. (2014). Superintelligence. Oxford: Oxford University Press.
Fuller, S. and Lipinska, V. (2014). The Proactionary Imperative. London: Palgrave (pp. 35–36).
Gerschenkron, A. (1962). Economic Backwardness in Historical Perspective. Cambridge MA: Harvard University Press.
Kahn, H. (1960). On Thermonuclear War. Princeton: Princeton University Press.
In professional cycling, it’s well known that a pack of 40 or 50 riders can ride faster and more efficiently than a single rider or small group. As such, you’ll often see cycling teams with different goals in a race work together to chase down a breakaway before the finish line.
This analogy is one way to think about collaborative multi-agent intelligent systems, which are poised to change the technology landscape for individuals, businesses, and governments, says Dr. Mehdi Dastani, a computer scientist at Utrecht University. The proliferation of these multi-agent systems could lead to significant systemic changes across society in the next decade.
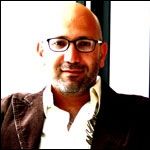
“Multi-agent systems are basically a kind of distributed system with sets of software. A set can be very large. They are autonomous, they make their own decisions, they can perceive their environment, “Dastani said. “They can perceive other agents and they can communicate, collaborate or compete to get certain resources. A multi-agent system can be conceived as a set of individual softwares that interact.”
As a simple example of multi-agent systems, Dastani cited Internet mail servers, which connect with each other and exchange messages and packets of information. On a larger scale, he noted eBay’s online auctions, which use multi-agent systems to allow one to find an item they want to buy, enter their maximum price and then, if needed, up the bid on the buyer’s behalf as the auction closes. Driverless cars are another great example of a multi-agent system, where many softwares must communicate to make complicated decisions.
Dastani noted that multi-agent systems dovetail nicely with today’s artificial intelligence. In the early days of AI, intelligence was a property of one single entity of software that could, for example, understand human language or perceive visual inputs to make its decisions, interact, or perform an action. As multi-agent systems have been developed, those single agents interact and receive information from other agents that they may lack, which allows them to collectively create greater functionality and more intelligent behavior.
“When we consider (global) trade, we basically define a kind of interaction in terms of action. This way of interacting among individuals might make their market more efficient. Their products might get to market for a better price, as the amount of time (to produce them) might be reduced,” Dastani said. “When we get into multi-agent systems, we consider intelligence as sort of an emergent phenomena that can be very functional and have properties like optimal global decision or situations of state.”
Other potential applications of multi-agent systems include designs for energy-efficient appliances, such as a washing machine that can contact an energy provider so that it operates during off-peak hours or a factory that wants to flatten out its peak energy use, he said. Municipal entities can also use multi-agent systems for planning, such as simulating traffic patterns to improve traffic efficiency.
Looking to the future, Dastani notes the parallels between multi-agent systems and Software as a Service (SaaS) computing, which could shed light on how multi-agent systems might evolve. Just as SaaS combines various applications for on-demand use, multi-agent systems can combine functionalities of various software to provide more complex solutions. The key to those more complex interactions, he added, is to develop a system that will govern the interactions of multi-agent systems and overcome the inefficiencies that can be created on the path toward functionality.
“The idea is the optimal interaction that we can design or we can have. Nevertheless, that doesn’t mean that multi-agent systems are by definition, efficient,” Dastani said. “We can have many processes that communicate, make an enormous number of messages and use a huge amount of resources and they still can not have a sort of interesting functionality. The whole idea is, how can we understand and analyze the interactions? How can we decide which interaction is better than the other interactions or more efficient or more productive?”