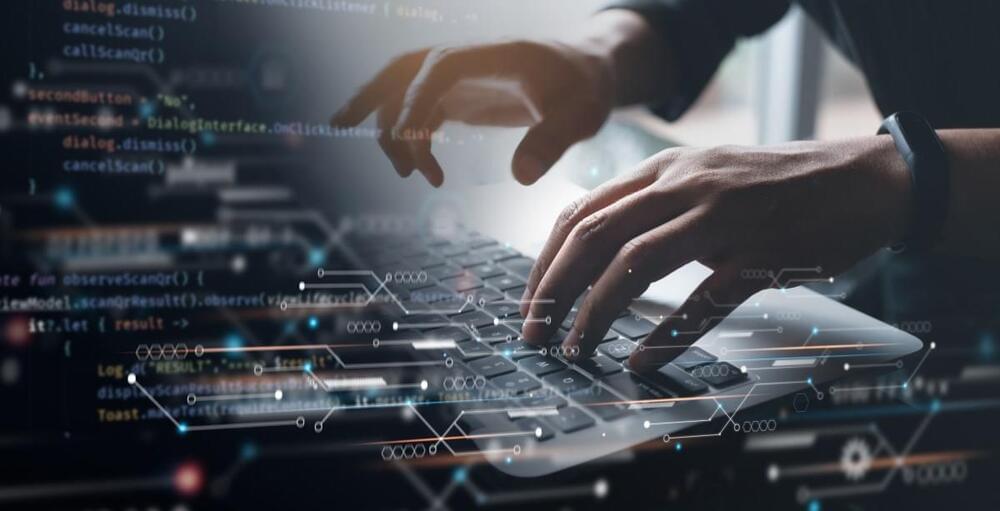
face_with_colon_three Basically although some or all coding jobs could be absorbed I remain positive because now everyone be a god now when infinite computation comes out and also infinite agi.
Jay Hack, an AI researcher with a background in natural language processing and computer vision, came to the realization several years ago that large language models (LLMs) — think OpenAI’s GPT-4 or ChatGPT — have the potential to make developers more productive by translating natural language requests into code.
After working at Palantir as a machine learning engineer and building and selling Mira, an AI-powered shopping startup for cosmetics, Hack began experimenting with LLMs to execute pull requests — the process of merging new code changes with main project repositories. With the help of a small team, Hack slowly expanded these experiments into a platform, Codegen, that attempts to automate as many mundane, repetitive software engineering tasks as possible leveraging LLMs.
“Codegen automates the menial labor out of software engineering by empowering AI agents to ship code,” Hack told TechCrunch in an email interview. “The platform enables companies to move significantly quicker and eliminates costs from tech debt and maintenance, allowing companies to focus on product innovation.”